How Monte Carlo Simulation Guides Decision-Making
Operational risk in financial institutions can emerge from unexpected corners, with one of the most severe examples being rogue trading. A single unauthorized trade can spiral into catastrophic losses, especially when factors like market volatility and leverage come into play. In this context, Monte Carlo simulation proves to be an invaluable tool, offering insights into potential risks, helping institutions prepare for worst-case scenarios, and making informed decisions to mitigate these risks.
In this article, we explore how Monte Carlo simulation can help financial institutions quantify and manage the risks associated with rogue trading, using a real-world scenario focused on unauthorized bond trading at a mid-sized UK bank.
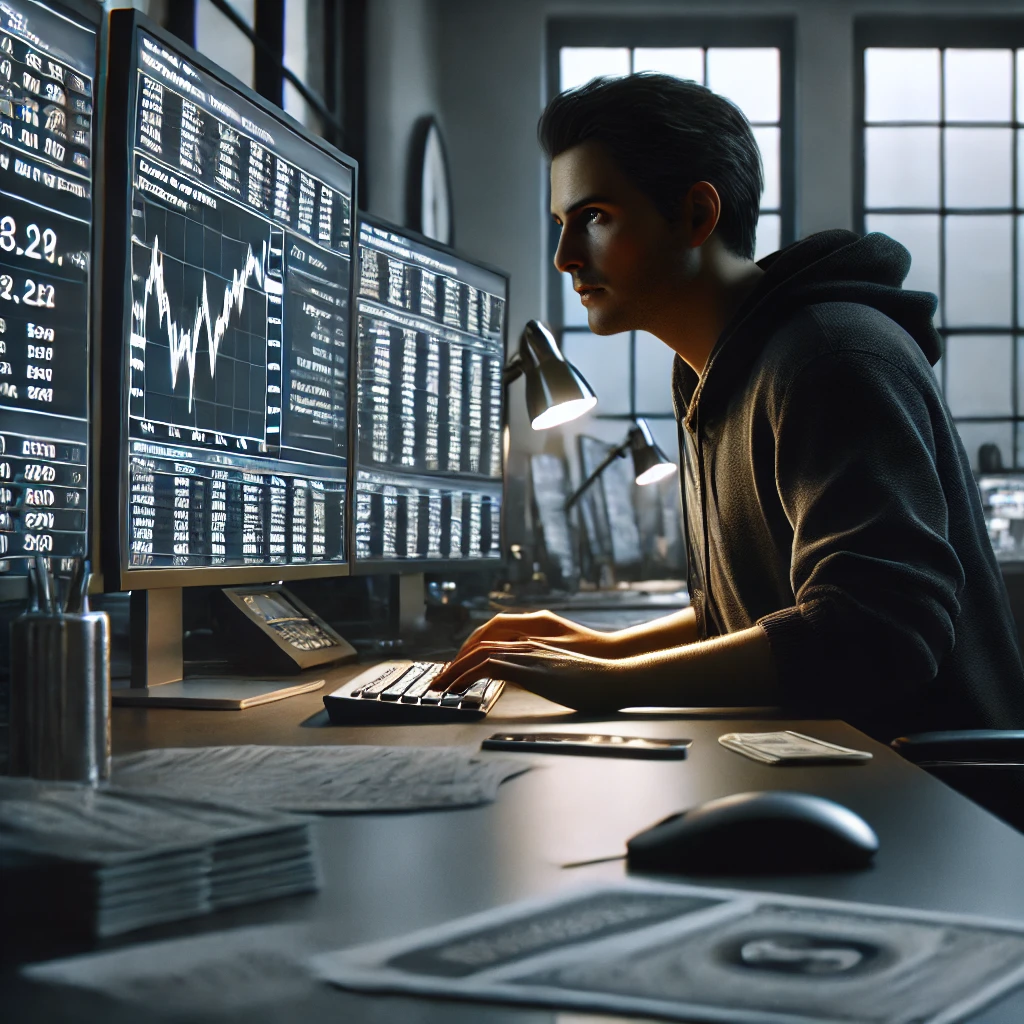
The Rogue Trading Scenario: Complex Risks with Severe Consequences
In this scenario, a rogue trader on a fixed-income desk engages in unauthorized bond trading, taking highly leveraged positions. The situation worsens when adverse interest rate movements, credit downgrades, and forced liquidation lead to escalating losses. This underscores the critical role of risk oversight and the devastating impact of hidden exposures.
But how can institutions foresee such complex risk dynamics? This is where Monte Carlo simulations become crucial. By modeling a wide range of possible outcomes—factoring in trade frequency, undetected periods, interest rate shocks, and market responses—Monte Carlo allows risk managers to quantify potential losses and develop strategies to address them.
How Monte Carlo Simulation Supports Decision-Making
1. Capturing the Full Spectrum of Risk
In rogue trading scenarios, many factors influence potential losses, from how long unauthorized trades remain undetected to the size of interest rate shocks and credit downgrades. Monte Carlo simulation captures the variability across these dimensions, generating thousands of possible outcomes based on different combinations of these variables. This gives decision-makers a clearer picture of not just the likely outcomes but also the extreme cases that could lead to severe financial exposure.
For instance, the simulation models key parameters such as:
- The frequency of unauthorized trades.
- The undetected trading period.
- Interest rate shifts and their impact on bond prices.
- Leverage ratios, which amplify both gains and losses.
- The possibility of credit downgrades affecting bond positions.
By integrating these variables, the simulation provides a holistic view of the potential exposure, from common scenarios to rare, catastrophic losses.
2. Quantifying Rare but High-Impact Events
One of the greatest benefits of Monte Carlo simulation is its ability to help businesses prepare for extreme but rare events. In the rogue trading scenario, adverse events such as interest rate shocks and credit downgrades have low probabilities but can lead to substantial losses when they do occur. The simulation quantifies these tail risks, giving risk managers data on how severe the impact could be in a 1-in-20 or 1-in-200 scenario.
For example, if interest rates shift by an unexpected margin, the simulation shows the effect on unauthorized leveraged bond positions. The outcomes from this simulation provide answers to critical questions: How much could we lose in a worst-case interest rate shift? What happens if a significant portion of unauthorized trades are downgraded in credit quality?
By offering probabilities attached to these extreme scenarios, Monte Carlo simulation gives institutions the foresight to prepare for the unexpected.
3. Understanding the Impact of Leverage
In financial markets, leverage is a double-edged sword—it magnifies gains but also amplifies losses. In this scenario, the rogue trader’s use of leverage multiplies the potential damage from unauthorized trades. The Monte Carlo simulation helps quantify just how much leverage could increase exposure to loss. It models different leverage ratios and shows how each increment could escalate financial risk, particularly in combination with market events like interest rate shifts or credit downgrades.
Through the simulation, institutions can see the compounded effects of leverage, making it easier to set limits or design policies to restrict unauthorized leverage usage. This is crucial because excessive leverage often turns what might have been a manageable loss into a disaster.
4. Measuring Combined Risk Exposures
Rogue trading risk doesn’t stem from a single factor—it’s a combination of market events (such as interest rate movements and yield curve shifts) and internal missteps (like undetected trades and excessive leverage). Monte Carlo simulation enables institutions to measure combined exposures by calculating how these various factors interact.
For instance, in this rogue trader scenario, the simulation evaluates:
- The effect of leveraged unauthorized trades on exposure.
- The impact of interest rate changes on those leveraged positions.
- Additional risks from yield curve shifts and credit downgrades.
The simulation also accounts for convexity adjustments—an additional cost incurred when unwinding bond positions in illiquid markets. All of these combined exposures can lead to total losses that are much larger than initially expected. By modeling these interactions, the Monte Carlo simulation reveals the potential for severe losses beyond what simple risk metrics might suggest.
5. Preparing for Regulatory and Market-Based Metrics
Finally, Monte Carlo simulations can inform regulatory stress testing by showing if an institution’s total exposure breaches critical thresholds under extreme conditions. For example, in this scenario, the simulation tracks whether exposure exceeds £12 million in a 1-in-20 event or £30 million in a 1-in-200 event—key metrics that would trigger regulatory or market-based concerns. This insight helps financial institutions comply with stress testing requirements while also giving them the opportunity to adjust their risk management strategies proactively.
Conclusion: Monte Carlo Simulation as a Strategic Risk Management Tool
In scenarios like rogue trading, where the interplay of unauthorized activity, market volatility, and leverage creates a web of risk, Monte Carlo simulation provides a clear framework for navigating uncertainty. By generating a range of possible outcomes, this tool helps financial institutions quantify both common and extreme risks, supporting data-driven decision-making that mitigates potential losses.
As financial markets become more complex and interconnected, the importance of understanding and managing operational risks cannot be overstated. Whether facing rogue traders or market shocks, Monte Carlo simulations offer a critical lens through which institutions can prepare for the worst while optimizing their strategies for the best outcomes.
Incorporating such simulations into your operational risk management approach today could be the key to avoiding tomorrow’s financial disaster.