Preparing for the Unexpected: Insights from a Monte Carlo Simulation
In financial services, operational resilience isn’t just a goal—it’s a requirement. Operational disruptions carry both financial and reputational costs, and senior management is tasked with minimising these risks while adhering to stringent regulatory expectations. Consider a scenario in which a bank’s data center cooling system fails, leading to an emergency shutdown of its loan processing platform. Suddenly, clients are unable to submit loan applications, and existing loans are left in limbo, with approvals and updates frozen. Costs begin to accumulate, from lost revenue to the operational burden of handling transaction backlogs and potential client compensation.
Monte Carlo simulations offer risk managers a powerful way to visualise and quantify the range of potential impacts of such incidents. Beyond averages, these simulations reveal the probabilities of various outcomes, enabling financial leaders to grasp the full scope of financial, operational, and regulatory consequences of a cooling system failure. Armed with these insights, decision-makers can better prepare, ensuring they have both the strategies and resources to effectively manage disruptions.
A Closer Look: Downtime and Transaction Backlogs
A critical cooling failure isn’t just a technical issue; it’s the first domino in a series of cascading effects that may disrupt client services, daily operations, and regulatory compliance. In this scenario, Monte Carlo simulations estimate an average downtime of around six hours. However, this could range from a quick two-hour fix to over ten hours in a worst-case scenario, accounting for the time needed to diagnose, repair, and bring the system back online.
This downtime isn’t just about the clock ticking—it translates into hundreds of unprocessed transactions. The simulation suggests that each hour of downtime leads to a backlog of 101 loan transactions, accumulating to an average of 601 unprocessed applications in the typical scenario. But in severe cases, the backlog could exceed 1,200 transactions, with a 12.9% chance of surpassing the critical threshold of 1,000. For risk managers, this insight is vital. Regulatory mandates often require incident reporting or increased oversight once impacted client numbers cross specific thresholds, such as 1,000. Knowing the likelihood of reaching these levels helps the bank develop preemptive policies for client communications, regulatory reporting, and service prioritisation during crises.
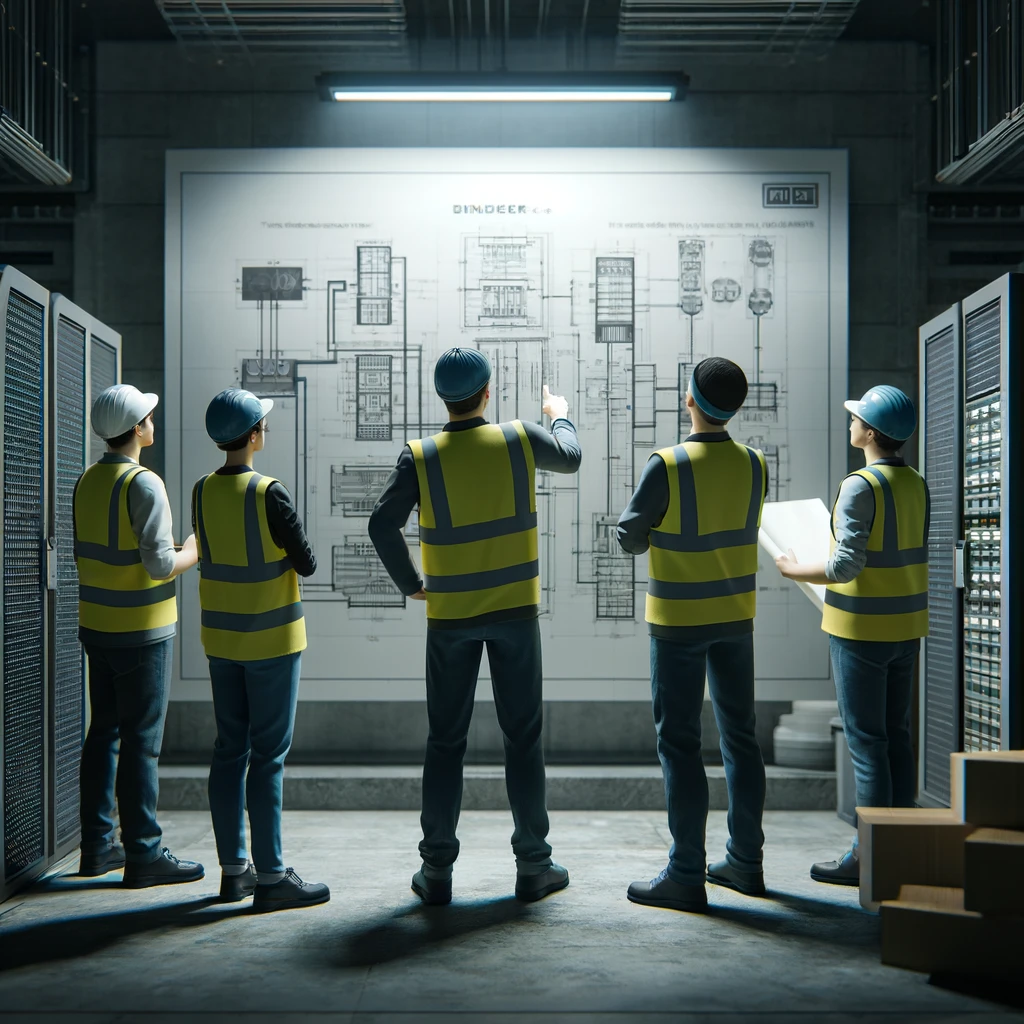
Financial Repercussions: Revenue Loss, Data Restoration, and Client Compensation
The financial costs of a cooling system failure are among the most immediate and tangible consequences. Each unprocessed loan transaction represents approximately £400 in lost revenue, and over a six-hour average downtime, this loss adds up to about £241,000. In severe scenarios, however, missed revenue can surpass £500,000. For management, understanding this range of potential losses highlights the urgency of rapid response to minimise downtime and restore operations.
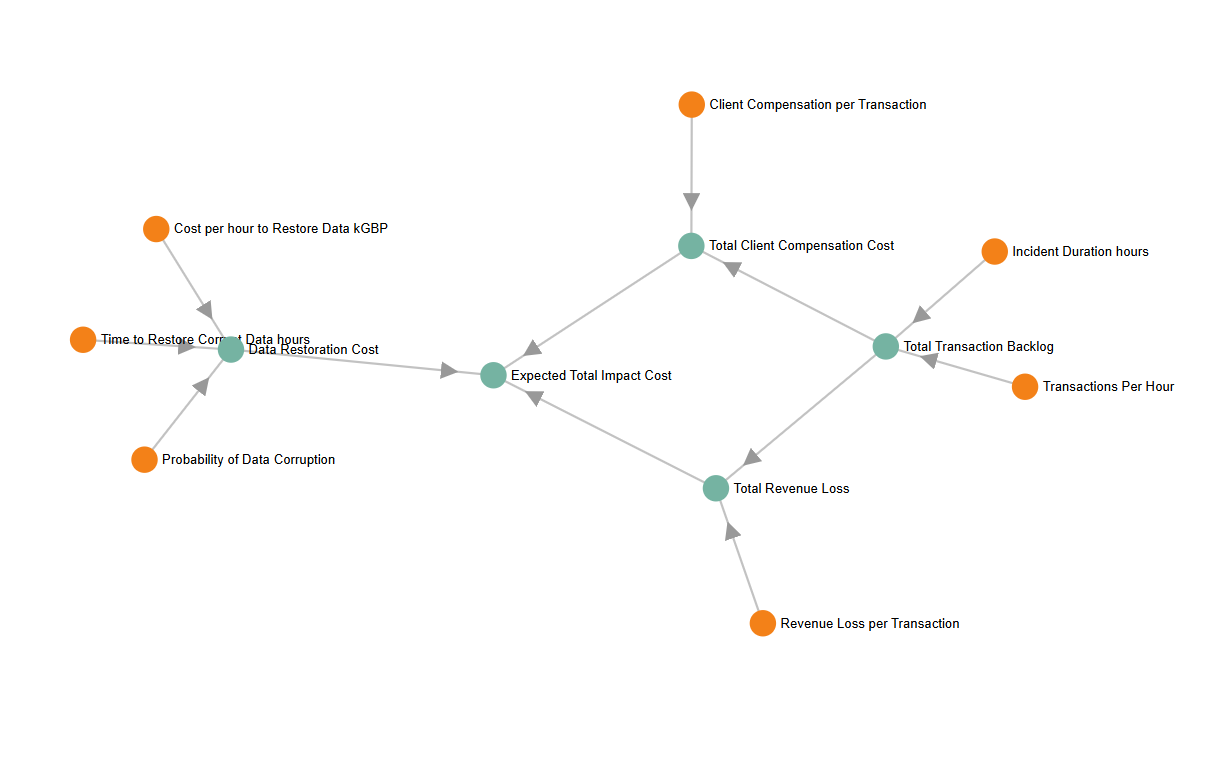
Data restoration costs add another layer of financial exposure. While data corruption is a relatively low-probability event (29.9%), the associated costs are high if it does occur. Restoration efforts—encompassing data integrity checks and verifications—carry an average cost of £5,931, though this could escalate to £14,000 in severe cases. Monte Carlo simulations are invaluable here as they capture the likelihood and potential impact of such discrete, high-cost events that, while not always occurring, carry significant consequences if they do.
Compensation for client inconvenience further adds to the financial toll. The simulation estimates an average compensation cost of £100 per affected transaction, resulting in an overall payout of around £60,000. However, high-end scenarios could drive this up to £145,000. This clarity around compensation helps management allocate funds more accurately; with an 84% probability that £200,000 would cover all compensation needs, the bank can align its budgets with modelled risk levels, meeting client expectations without excessive over-allocation.
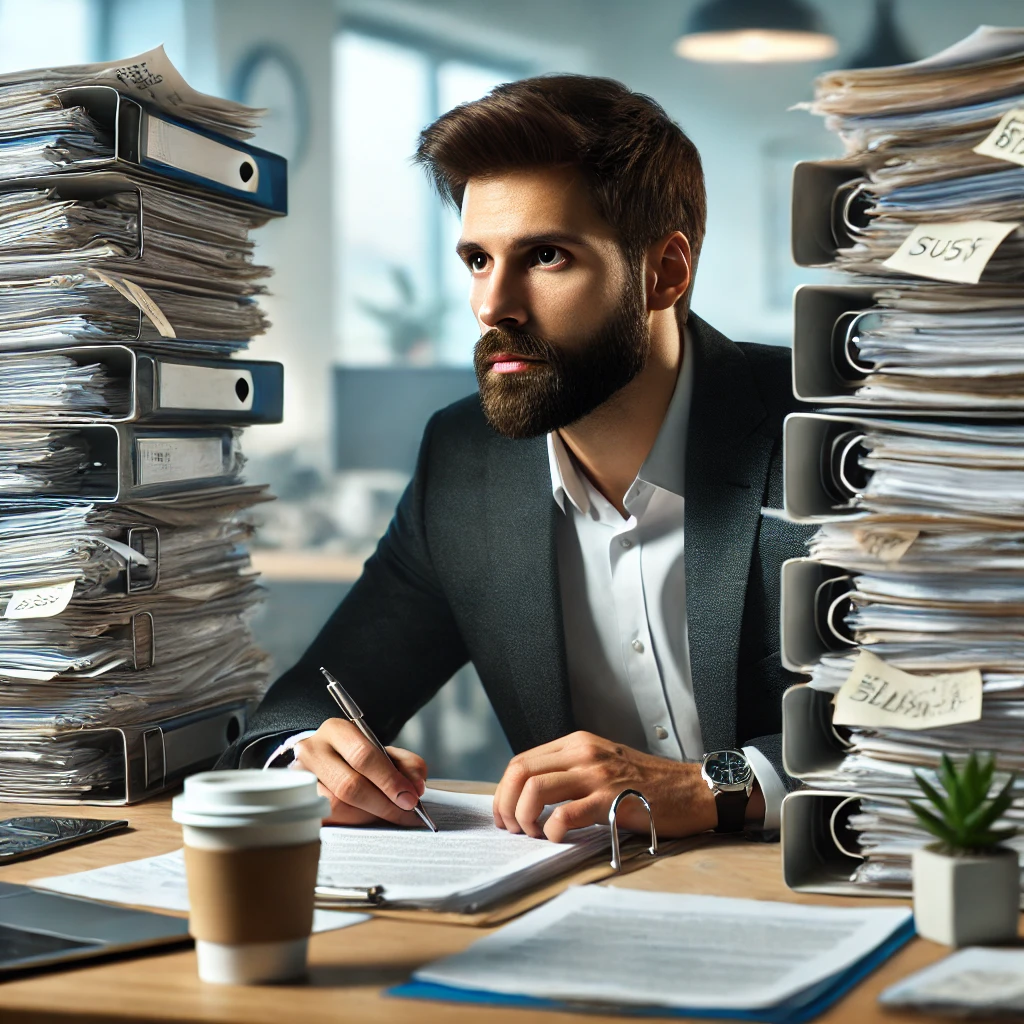
Expected Total Impact Cost: A Comprehensive Financial Exposure
Bringing all these factors together, the simulation reveals an expected total impact cost of £306,000, though worst-case scenarios could see this reaching £645,000. Crucially, the simulation shows only a 0.24% probability that total costs could exceed £1 million—an insight with real regulatory implications. This aligns with operational risk capital requirements, particularly the need to hold capital against rare, extreme events. By knowing the likelihood of such an event surpassing £1 million, the bank can ensure it remains appropriately capitalised.
Adapting to Changing Assumptions: A Key Advantage of Monte Carlo Simulation
One of the major strengths of Monte Carlo simulation is its capacity to swifty reflect changes to underlying assumptions. This flexibility became especially valuable when management requested a shift from average transaction volumes to a focus on a peak transaction period, such as early spring, when loan processing demand typically increases in line with the start of home owner renovation projects such as installing a new kitchen. By adjusting the model to reflect this busy period, the simulation delivered a more realistic view of potential impacts, revealing significant differences that might have been overlooked with generalised assumptions.
For example, during the busy period, the projected transaction backlog almost doubled. While the original average-case scenario estimated an average backlog of around 601 transactions, the busy-period adjustment raised this figure to 1,185 transactions. In high-end scenarios, the backlog rose from a previous maximum of 1,200 transactions to over 2,400. Additionally, the probability of exceeding the regulatory notification threshold of 1,000 impacted transactions rose sharply—from 12.9% to 55.7%. This insight is crucial for management, as higher transaction volumes during peak times mean a significantly increased likelihood of mandatory incident reporting and potential regulatory scrutiny.
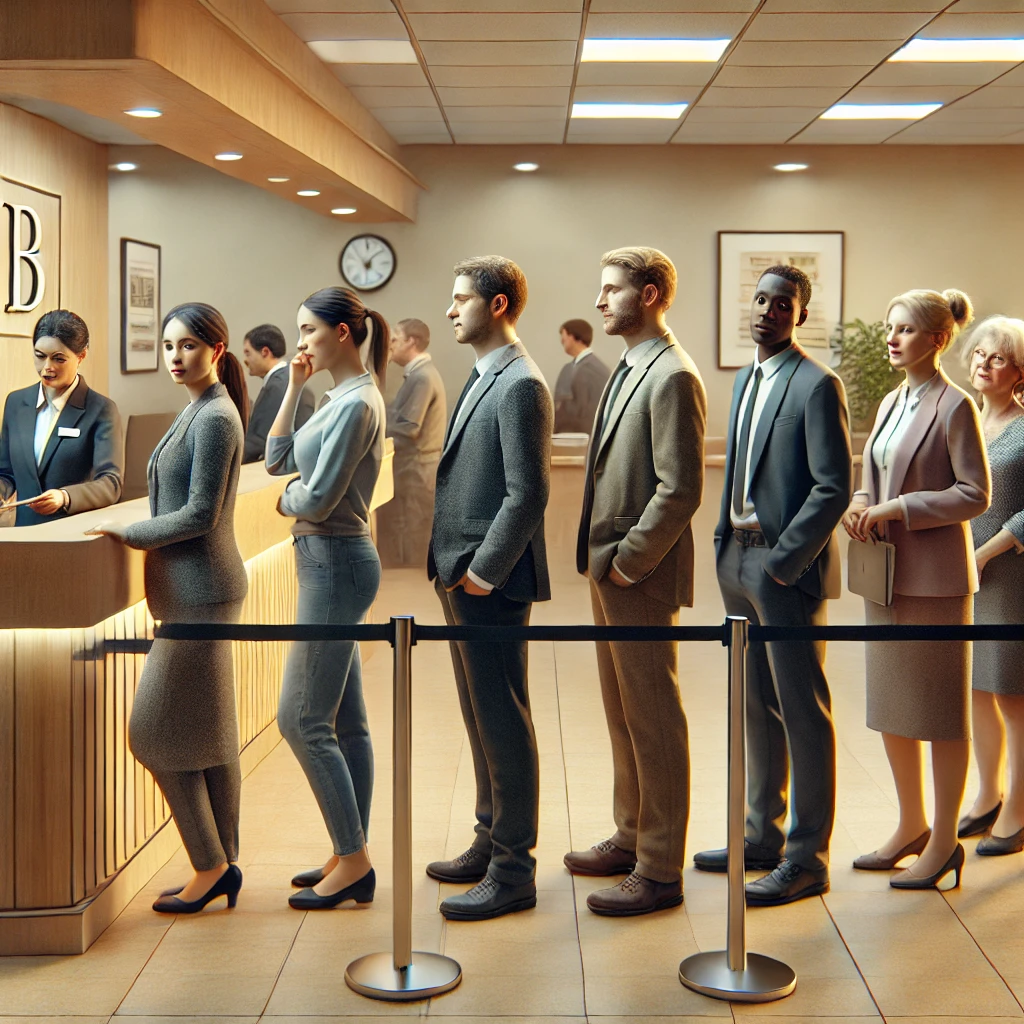
The financial impacts saw similarly notable changes. For instance, the estimated revenue loss during a busy period disruption was much higher, with an average loss increasing from £241,000 to £473,000, and high-end losses reaching up to £990,000—nearly double the initial high-end projection. The likelihood that a £200,000 compensation budget would cover client inconvenience costs also dropped considerably, from 84% to 49%, indicating that reserves may need adjusting to meet the demands of peak periods.
This adaptability of Monte Carlo simulations allows risk managers to challenge and refine initial assumptions easily, testing different scenarios to ensure that operational resilience planning is robust under varying business conditions. By accommodating shifts in key assumptions, the simulation provides a more nuanced and relevant view of potential outcomes, increasing confidence in the bank’s preparedness for both typical and high-demand periods. This flexible, iterative approach empowers financial institutions to optimise resilience strategies and regulatory responses, ensuring they are better equipped to handle both average and high-stress conditions effectively.
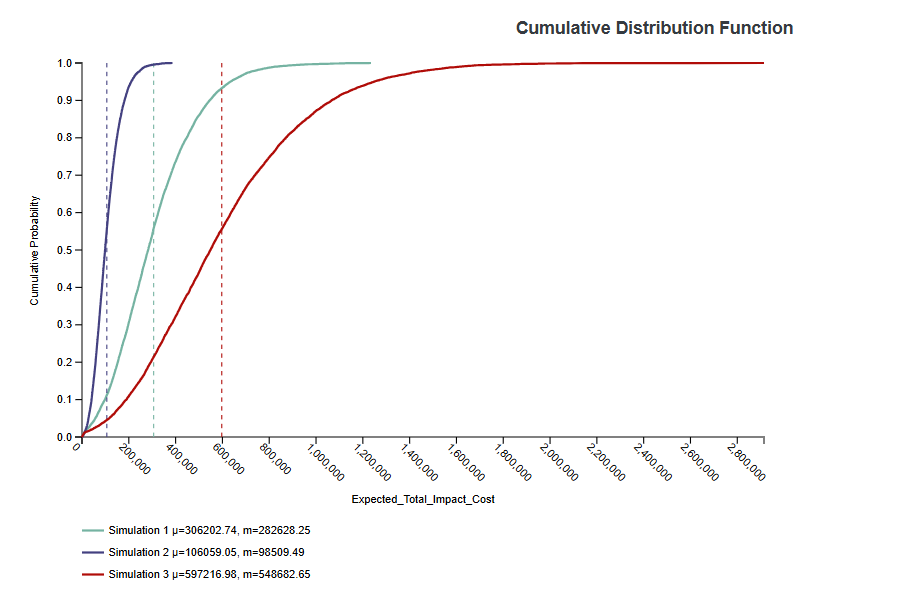
A Closer Look: Incident Duration and Transaction Backlog
The simulation might reveal that, without any mitigating actions, the average downtime due to a cooling failure is around six hours, with a range spanning from two to over ten hours. Each hour of downtime results in approximately 100 unprocessed transactions, leading to a potential backlog of 600 transactions on average.
Now, suppose the bank evaluates the impact of installing a redundant cooling system, which could reduce the average downtime to just two hours. The simulation would show a significant decrease in transaction backlogs and associated costs, providing a compelling case for the investment.
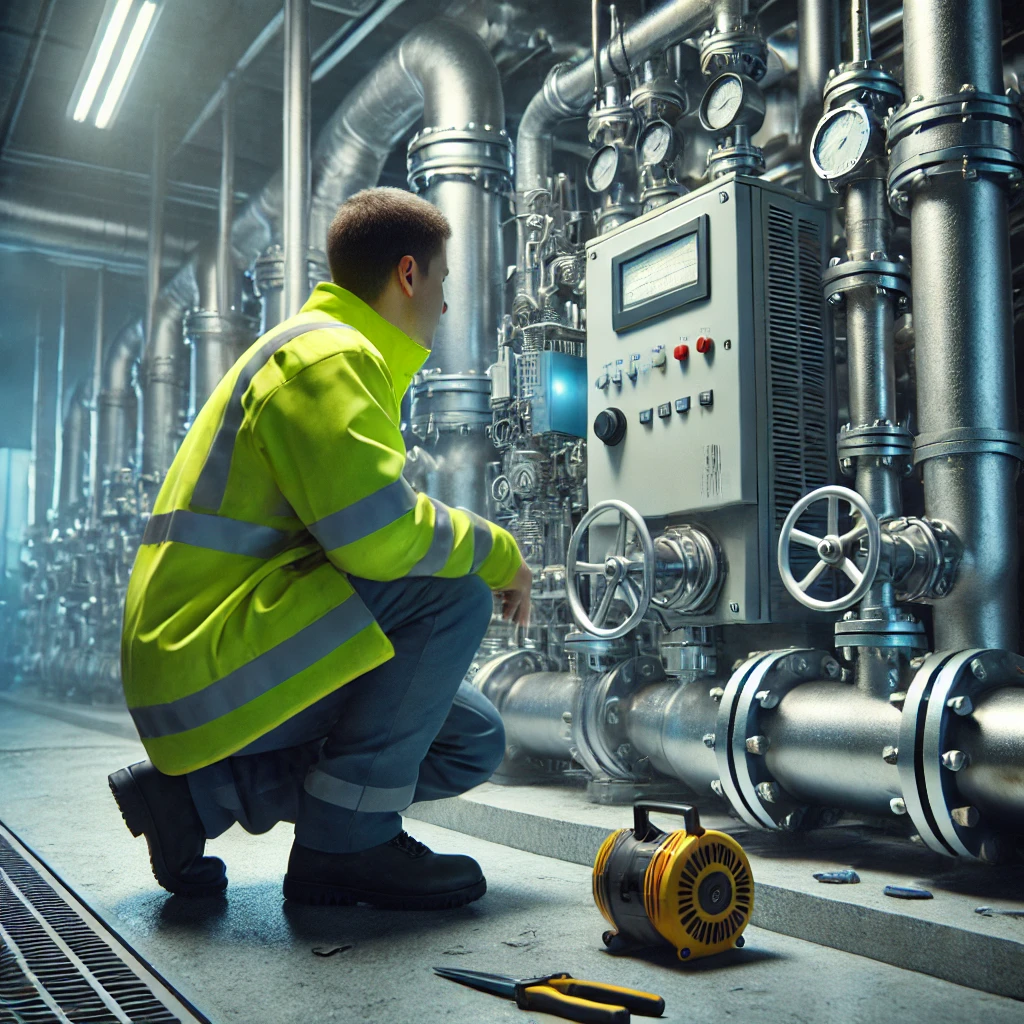
Strategic Value of Monte Carlo Simulation for Risk Management
Monte Carlo simulations excel in risk management because they deliver a full distribution of potential outcomes rather than just a point estimate. This granularity empowers senior management to grasp both typical and extreme scenarios, enabling proactive preparation for high-impact, low-likelihood events. The ability to assess discrete risks—such as data corruption—adds valuable depth to risk analyses, allowing the bank to target specific areas, like data protection, where further investment may be beneficial.
As regulatory requirements around capital reserves and incident reporting evolve, Monte Carlo simulations offer decision-makers a quantitative tool to meet these standards. By mapping potential outcomes, senior leaders gain a clearer view of where investments in resilience, compensation policies, and business continuity planning will yield the highest returns. This data-driven approach not only helps optimise risk response but also reinforces operational resilience, ensuring that even in the face of worst-case scenarios, banks are well-equipped to manage both financial and regulatory challenges effectively.
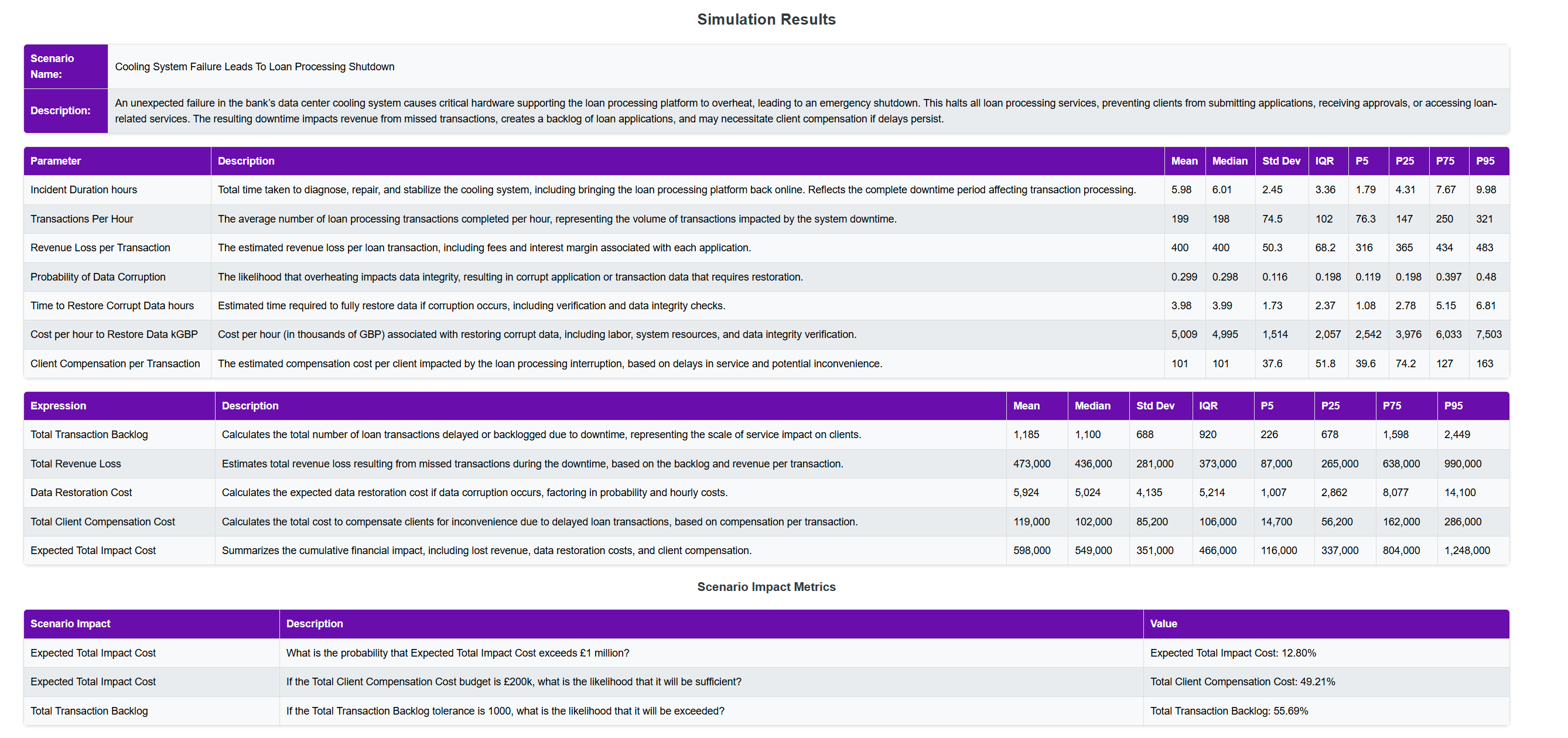