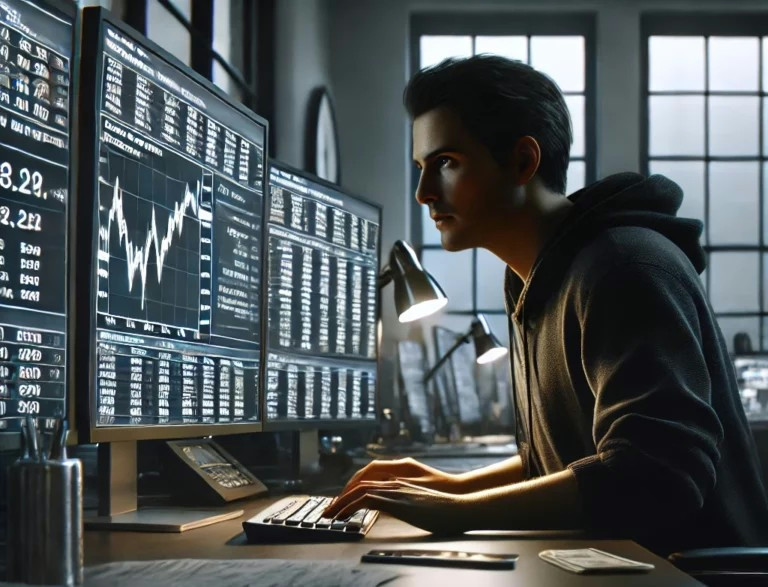
Scenario Overview:
In this scenario, a rogue trader on a fixed-income desk at a mid-sized UK bank engages in unauthorised bond trading, taking leveraged positions as they seek to magnify the gains. The situation worsens when adverse interest rate movements, credit downgrades, and forced liquidation lead to escalating losses.
The scenario reveals a critical blind spot in operational risk management: the difference between additive and multiplicative risk assessments. Many approaches simply add up best guesses of potential impacts—the predicted loss plus some regulatory fine plus the cost of a skilled persons review (S166). But reality is rarely this linear. In many scenarios, risk factors multiply instead: the scale of loss is amplified by the duration of the incident and the impact of external events. Each factor doesn’t add to the total—it multiplies it. Let’s examine the key parameters that create this multiplier effect.
Key Parameters and What They Mean
- Unauthorised Trade Frequency The number of unauthorised trades per week. Even a modest frequency can build a substantial exposure if left undetected.
- Undetected Period How long these trades remain hidden. Crucial because the potential loss grows the longer the misconduct goes unnoticed.
- Undetected Trade Value The monetary size of each transaction. Scaled up by frequency and duration, it forms a base for subsequent losses.
- Leverage Ratio: The factor by which the rogue trader amplifies each position. Even small market shifts become severe when leverage is high.
Assessments and Expressions
The scenario analysis uses a set of formulas to estimate final exposure. These expressions demonstrate the multiplicative nature of certain risk drivers: a higher frequency, extended duration, and heavier leverage can turn a moderate event into a severe one—especially if interest rates move unfavourably and credit conditions worsen.
Scenario Impact Analysis
The analysis illustrates how outcomes might range from mild to extreme. In this scenario the mean value for potential exposure is around £1.8m, but with a 1-in-20 (P95) close to £6m, indicating that losses could balloon under adverse but plausible conditions, and a potential extreme but still plausible (1-in-200) exposure of £14m. The impact of events such as Interest Rate movements or and Credit Downgrades vary widely, from near zero at the low end (if market or credit shifts are minimal) up to multiple times the base value at the higher percentiles. Taken together, these parameters show how a small number of hidden trades in a short window might remain manageable—yet a longer detection gap or larger trade sizes, when amplified by external events, can push potential losses significantly higher. Furthermore, even moderate leverage multiples can sharply exacerbate the loss range once markets move.
By examining these distribution ranges rather than a single point estimate, risk professionals see how losses might stack up under less likely but still credible circumstances.
Why This Matters for Operational Risk
Quantifying Rare but High-Impact Events
Time as a Multiplier The longer unauthorised activity persists, the higher the potential for compounding damage. This underscores the critical importance of detection controls along side preventative ones.
Quantifying rare but high-impact events Scenario analysis can help businesses prepare for extreme but rare events. Monte Carlo simulation quantifies these tail risks, giving risk managers insight on how severe the impact could be in a 1-in-20 or 1-in-200 scenario.
Communicating Uncertainty: Being able to discuss distribution percentiles helps explain why certain additional controls or investigations are justified. It also gives boards and senior management a concrete understanding of what “severe loss” could look like.
Beyond Basic Tools Scenario-based methods and distributions reveal real tail events in a way a simple risk matrix or single “worst case” figure cannot. For managers, it’s a powerful reminder that improbable does not equal impossible.
Takeaways
- Multiple Risk Drivers: No single factor alone (such as interest rate movement) tells the full story; it’s the interplay of risk factors, time and events that shapes the risk. Understanding these dynamics should be a central pillar of developing resilience.
- Quantifying ‘What If?’: By gauging a range of outcomes—expected, significant and extreme—operational risk managers can prioritise oversight and highlight potential high-impact exposures to stakeholders.
- Communicating Uncertainty: Being able to discuss distribution percentiles helps explain why certain additional controls or investigations are justified. It also gives boards and senior management a concrete understanding of what “severe loss” could look like.
- Strategic Prevention: Armed with these insights, organisations can allocate resources and implement controls that specifically target the drivers most likely to create outsize losses.
In short, this scenario illustrates of how multiple risk elements can compound. The time dimension and multiplicative effect of scale and external factors can magnify a seemingly small exposure into something significant. Scenario analysis and distribution-based approaches can transform abstract possibilities into concrete insights that drive better decision-making.
Want to see these principles in action? Visit RiskSpace.com to explore this scenario in an interactive Monte Carlo simulation. You can adjust the parameters yourself to understand how different factors multiply to create tail risks that traditional models might miss. Drop me a message if you’d like a walkthrough or to discuss your own scenario analysis challenges.