Inside a One-Hour Outage: Monte Carlo Simulation Reveals Risks and Resilience
Imagine it’s 9:15 on a bustling Tuesday morning at a mid-sized UK bank with £70 billion in assets. As employees settle into their tasks and customers log into their accounts, disaster strikes: the bank’s Identity and Access Management (IAM) system fails entirely. For the next hour, neither customers nor staff can authenticate into digital banking systems. This unexpected outage locks out 2 million customers and 12,000 employees, halting services that are vital to the bank’s day-to-day operations. While the issue lasts only an hour, the effects are anything but brief.
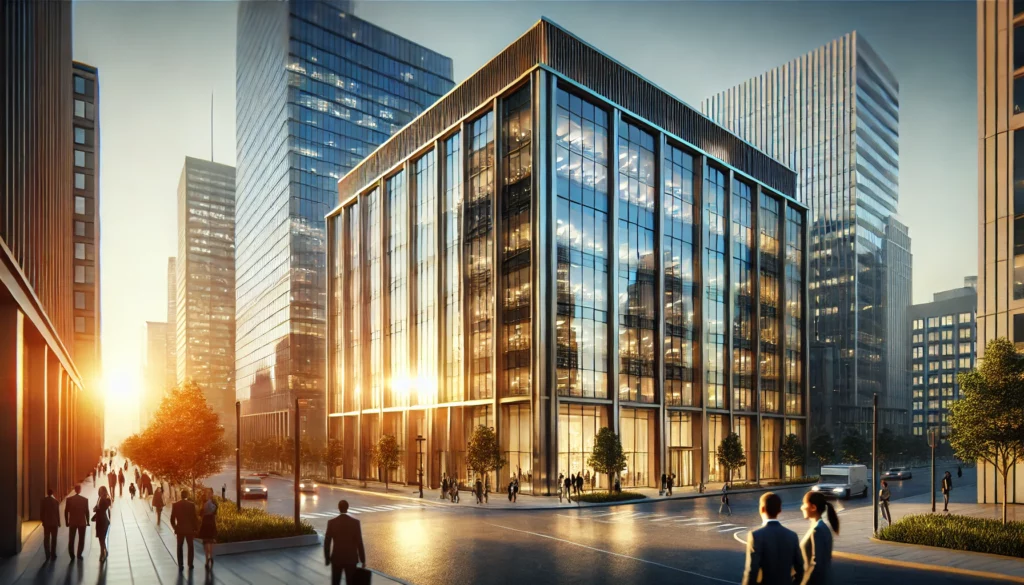
To understand the full scope of this risk, we used a Monte Carlo simulation to model thousands of potential outcomes based on real-world parameters. By doing so, the bank could quantify the impact of this one-hour outage across financial, operational, and customer service dimensions. This simulation reveals important insights into how an hour of downtime can cascade across an organisation, emphasising the importance of robust planning, both for restoring services and for managing the downstream effects.
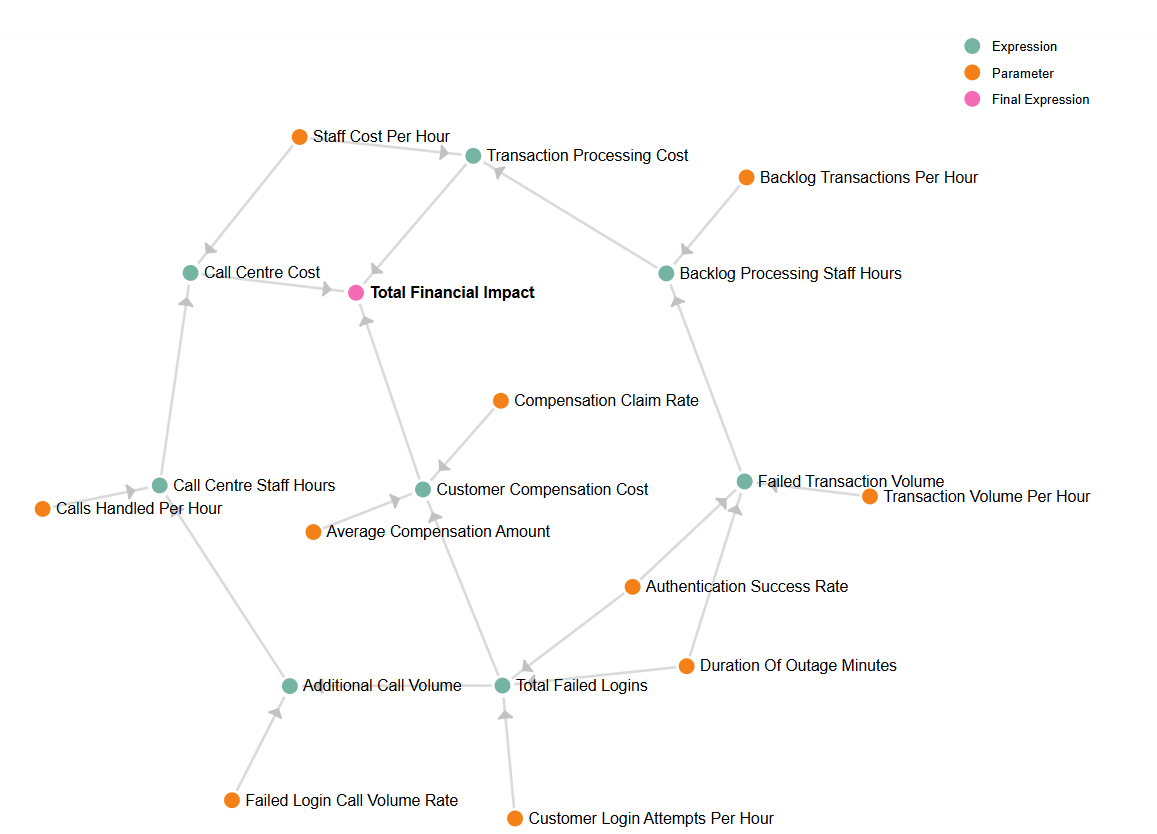
Financial Impact: Gauging the True Cost of Downtime
When IAM services fail, a bank’s financial exposure goes beyond immediate technical recovery costs. The simulation shows that on average financial losses would be around £300,000. This figure is derived from multiple sources of cost, including call center staffing, transaction backlog processing, and customer compensation payments. There is a unlikely scenario, one-in-20 outcomes, that the financial impact could reach £600,000, and for an even more extreme scenario — the financial impact exceeding £900,000 — the probability drops to 0.5%, equivalent to a 1-in-200 event. These probabilities give the bank perspective on the severity of the risk and highlight the need for preventative measures, such as investing in IAM system reliability and backup solutions.
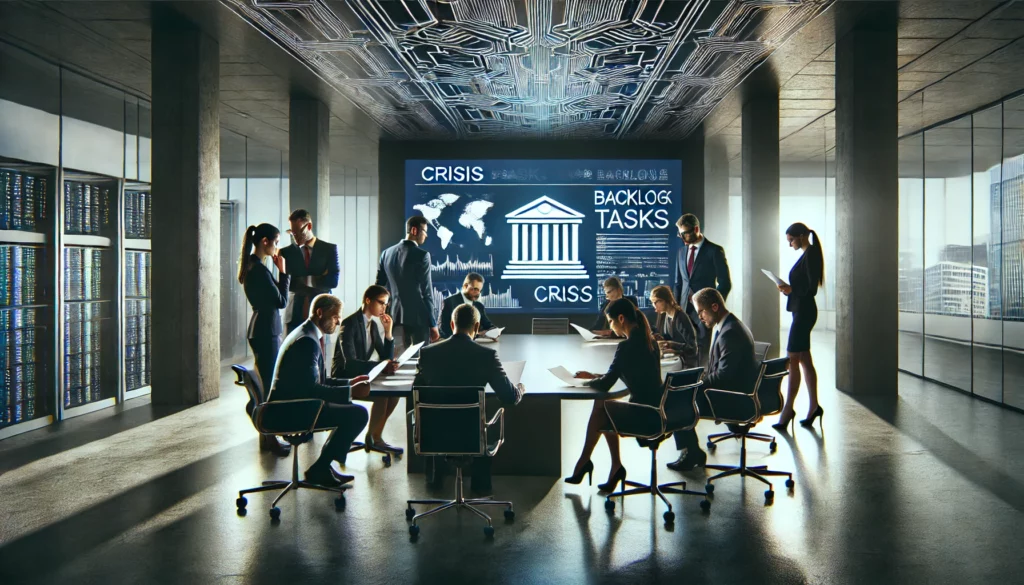
The primary driver of these costs is the volume of failed login attempts and subsequent customer support calls. During the outage, the bank would experience an estimated 80,000 login attempts per hour. With authentication completely disabled, all these attempts would fail, which leads directly into the next area of impact: customer support.
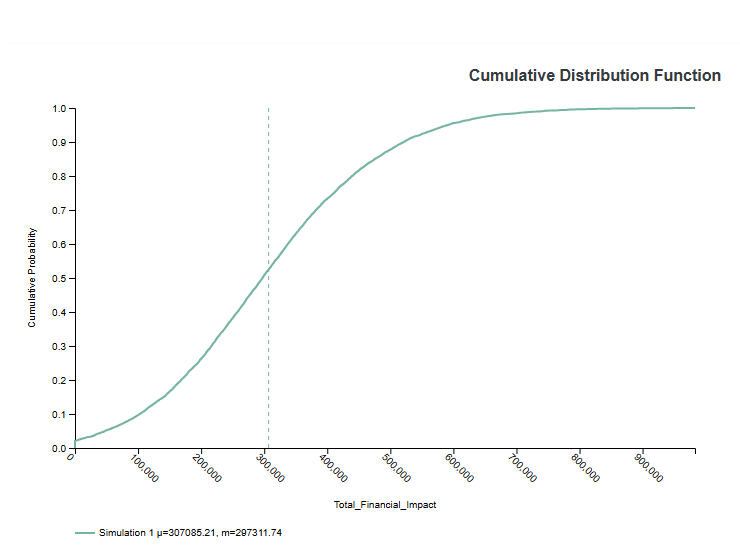
Customer Service Strain: Handling a Surge in Support Requests
Failed logins not only disrupt customer access but also create a cascade effect on the bank’s customer service resources. The model indicates that a large proportion of these failed logins would result in calls to the bank’s support center, especially as customers become frustrated with their inability to access accounts. According to the simulation, around 15% of failed login attempts are likely to generate a support call, resulting in over 12,000 additional calls during the outage. This sudden spike in call volume would require substantial staffing adjustments, potentially needing hundreds of additional call center hours just to handle the influx.
The model further estimates that the total number of call center staff hours required to meet this spike in demand would exceed 1000 hours. Without proper preparation, customers would face long wait times, leading to frustration and potential reputational damage. This underscores the need for banks to have flexible, surge-ready call center resources. Contingency planning for high-impact outages should consider not only the technical recovery process but also the ability to respond to customer needs in real-time, maintaining service standards in stressed conditions.
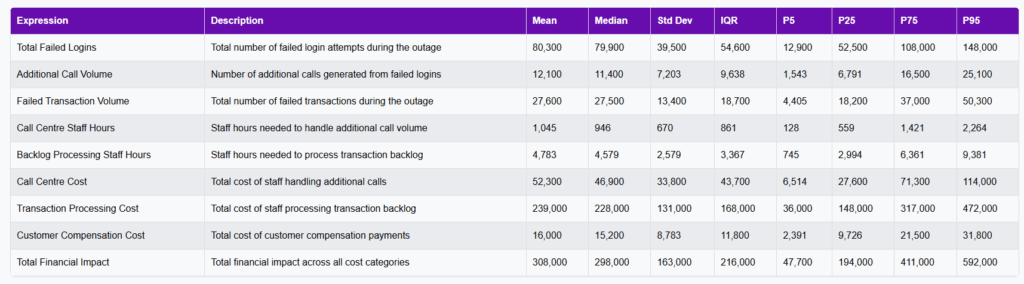
Operational Strain: Clearing the Transaction Backlog
An IAM outage also disrupts the bank’s internal operations, especially around transaction processing. With digital services offline, standard banking transactions—payments, transfers, deposits—are interrupted. The simulation reveals that every hour of disruption leaves behind a significant backlog of failed transactions, each requiring manual intervention to clear once the systems are back online.
In this scenario, the estimated backlog of failed transactions, based on normal transaction volumes of 50,000 per hour, is substantial and the simulation projects that clearing this backlog would require extensive staffing and add considerable operational costs. The burden of clearing transaction backlogs can persist for hours or even days after the initial outage, impacting productivity and workflow. This highlights the importance of having a rapid post-outage recovery plan, with processes in place to prioritise and address transaction backlogs efficiently.
Deeper Exploration of Financial Drivers in the IAM Outage
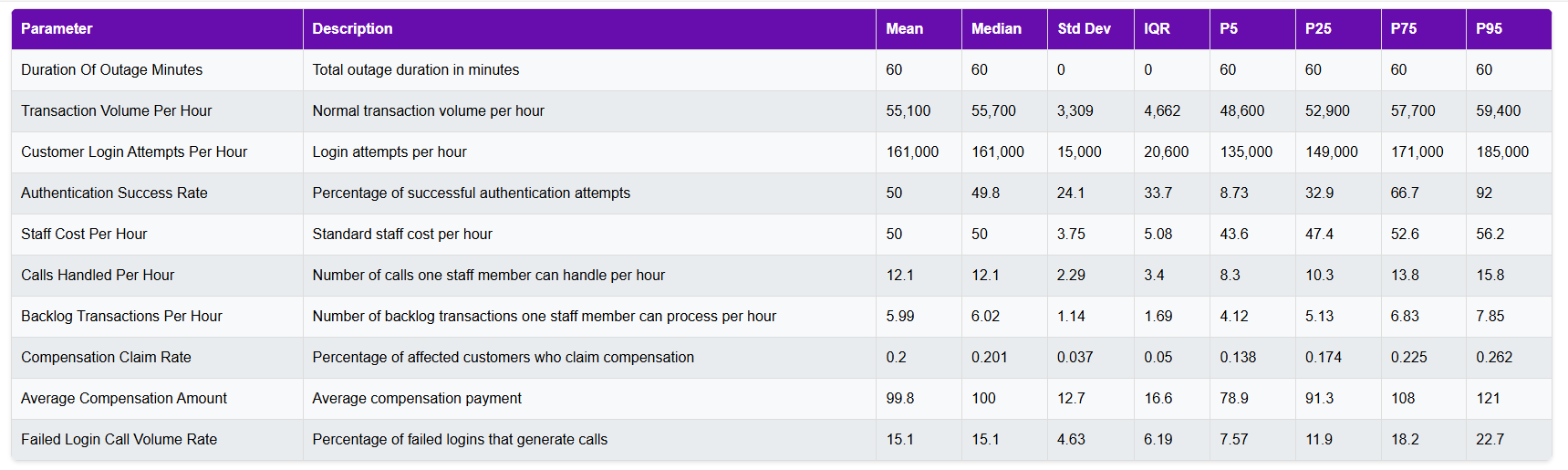
When considering the financial impact of a one-hour IAM outage, it’s helpful to break down the specific cost drivers involved, as each component plays a distinct role in the total potential loss. According to the Monte Carlo simulation, the main contributors to the financial impact include:
Call Center Costs: The surge in customer service calls resulting from failed logins is one of the largest direct costs. With an estimated 10,000 additional calls generated during the outage, the bank would need to deploy significant resources to handle the increased call volume. Staffing costs for the additional call center hours needed are projected to contribute substantially to the overall financial impact. If the bank is unable to quickly adjust staffing, these costs could rise even higher as wait times increase and customer satisfaction declines.
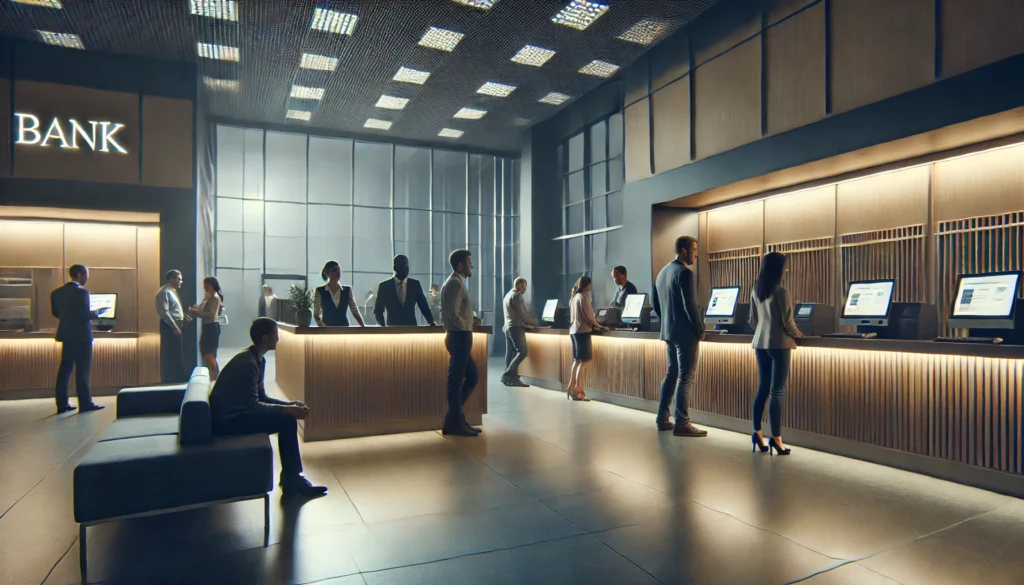
Transaction Processing Costs: Each failed transaction that occurs during the outage contributes to a backlog, requiring manual processing once systems are back online. In the scenario modeled, backlog processing would necessitate considerable staff hours, adding operational costs that extend beyond the outage itself. Since each staff member can only handle a limited number of backlog transactions per hour, this cost can scale quickly, especially if the backlog disrupts the bank’s regular transaction flow.
Customer Compensation Costs: The simulation estimates that around 0.1% of affected customers could file compensation claims due to the inconvenience or financial loss experienced during the outage. While this percentage seems small, it represents roughly 2,100 claims for a customer base of 2 million, with each payout averaging £50. While this may not be a primary driver, customer compensation remains a meaningful cost that can add up quickly, especially when considering both direct payouts and the administrative resources required to handle claims.
Together, these components—call center staffing, transaction backlog processing, and customer compensation—form a complex web of costs that the bank would need to address in an actual outage scenario. Understanding the breakdown allows the bank to focus its contingency planning on areas with the highest impact, ensuring that resources are allocated to the most pressing financial and operational needs during a crisis.
Beyond the Numbers: Strategic Insights for Risk Management
The insights from this simulation aren’t just theoretical; they provide actionable guidance for the bank’s risk management strategy. By analysing financial, operational, and customer service impacts, the bank can make more informed decisions on how to prepare for, mitigate, and respond to an IAM service outage.
First, the data highlights the value of investing in system redundancy and reliability for IAM services. Given the relatively low but substantial risk of severe financial impact, allocating resources to prevent or quickly recover from IAM failures can provide a strong return on investment.
Second, the findings point to the need for flexible, surge-ready customer support teams. Ensuring that additional call center resources can be mobilised quickly during a crisis is essential to maintaining service levels and customer satisfaction.
Finally, the operational insights around transaction backlogs underscore the importance of having a dedicated post-outage recovery process. This includes clear prioritisation of backlog transactions, efficient staffing plans, and perhaps automated tools to streamline the manual process.
Enhancing Risk Mitigation: Practical Strategies to Reduce Impact
The Monte Carlo simulation results highlight the significant strain an IAM outage could place on financial, operational, and customer-facing functions. Based on these insights, the bank could explore several practical mitigation strategies to minimise both the likelihood and impact of a future IAM outage:
Investing in System Redundancy: One of the most direct ways to prevent outages is by enhancing IAM system resilience. Implementing redundancy measures, such as backup servers, automated failover systems, and diversified network paths, can help ensure continuity even if the primary IAM system encounters issues. Regular testing of these systems is essential to ensure they work seamlessly during a real incident.
Developing a Surge Staffing Plan for Call Centers: Given the likelihood of a call volume spike, the bank could create a contingency plan to deploy additional call center staff at short notice. This might include cross-training employees or establishing partnerships with third-party customer service providers. By having a flexible staffing strategy, the bank can ensure it meets customer demand during high-impact events without compromising response times.
Implementing Automated Backlog Processing Tools: The operational impact of clearing transaction backlogs can be minimised with automation. Robotic Process Automation (RPA) tools, for instance, can assist in processing transactions more quickly and efficiently, reducing the manual workload on staff. By automating repetitive transaction handling tasks, the bank can clear backlogs faster and limit the disruption to daily operations.
Establishing a Customer Communication Protocol: During an outage, proactive communication is crucial for maintaining customer trust. The bank should have in place a pre-planned communication protocol that includes regular updates on service status, expected recovery times, and instructions on alternative service options. Transparent communication can help reduce frustration and potentially lower the number of customer service calls and compensation claims, as customers are kept informed of the situation.
These mitigation strategies represent a proactive approach to managing the risks of an IAM outage. By addressing both technical and operational contingencies, the bank can enhance its resilience and better safeguard customer relationships and financial stability in the face of unforeseen disruptions.
The Broader Value of Monte Carlo Simulations in Financial Services
In a world increasingly driven by digital services, Monte Carlo simulations are becoming essential tools for operational resilience. They allow banks to anticipate the potential outcomes of rare but impactful events, giving them a clearer picture of risks and required responses. As this scenario shows, the power of simulations lies in their ability to break down complex, interconnected risks—financial, operational, and customer-related—into actionable insights.
By proactively modeling various scenarios, banks can develop targeted strategies to mitigate disruptions, enhance customer service, and maintain operational continuity. In a highly competitive market, where both customers and regulators expect uninterrupted access to financial services, simulation-based risk management is not just a defensive strategy—it’s a crucial component of building resilience and trust.
For financial institutions and other sectors facing complex operational risks, Monte Carlo simulations offer a pathway to understanding and preparing for the uncertainties that come with digital dependency. Through data-driven insights, organisations can strengthen their defenses, ensuring they’re not only reactive but also resilient when the unexpected occurs.