System Crash, Compliance Risk, and Financial Fallout
In the interconnected world of financial services, operational disruptions can quickly cascade into compliance breaches, reputational damage, and substantial financial loss. Consider a scenario where a key third-party provider responsible for anti-money laundering (AML) transaction monitoring experiences a system outage. This results in a prolonged downtime, forcing the bank to review transactions over £2,000 through a semi-automatic in-house process, while transactions exceeding £10,000 are blocked for manual review.
While manual processing may serve as a temporary workaround, it introduces significant operational strain and the risk of errors. Worse yet, failure to detect suspicious activity or failure to correctly processing transactions could potentially lead to fines or reputational harm. To quantify this risk, we ran a Monte Carlo simulation that models potential outcomes based on key parameters such as downtime duration, transaction volume, and manual error rates. The results shed light on the depth of the problem and the financial exposure that such an outage could create for the bank.
Key Findings from the Simulation: Navigating the Risks of AML Downtime
Imagine it’s midday, and your bank’s third-party anti-money laundering (AML) system suddenly crashes. At first, this seems manageable thanks to robust continuity planning. The bank has a proportional, risk-based approach: transactions below £2,000 continue to be processed normally, with a post-event review in place to identify any suspicious activity. Transactions over £2,000 are routed through a semi-automatic in-house system, while those exceeding £10,000 are sent for manual review. The response helps, but as the outage stretches into a 36-hour downtime, the backlogs grow, mistakes happen and the the pressure intensifies.
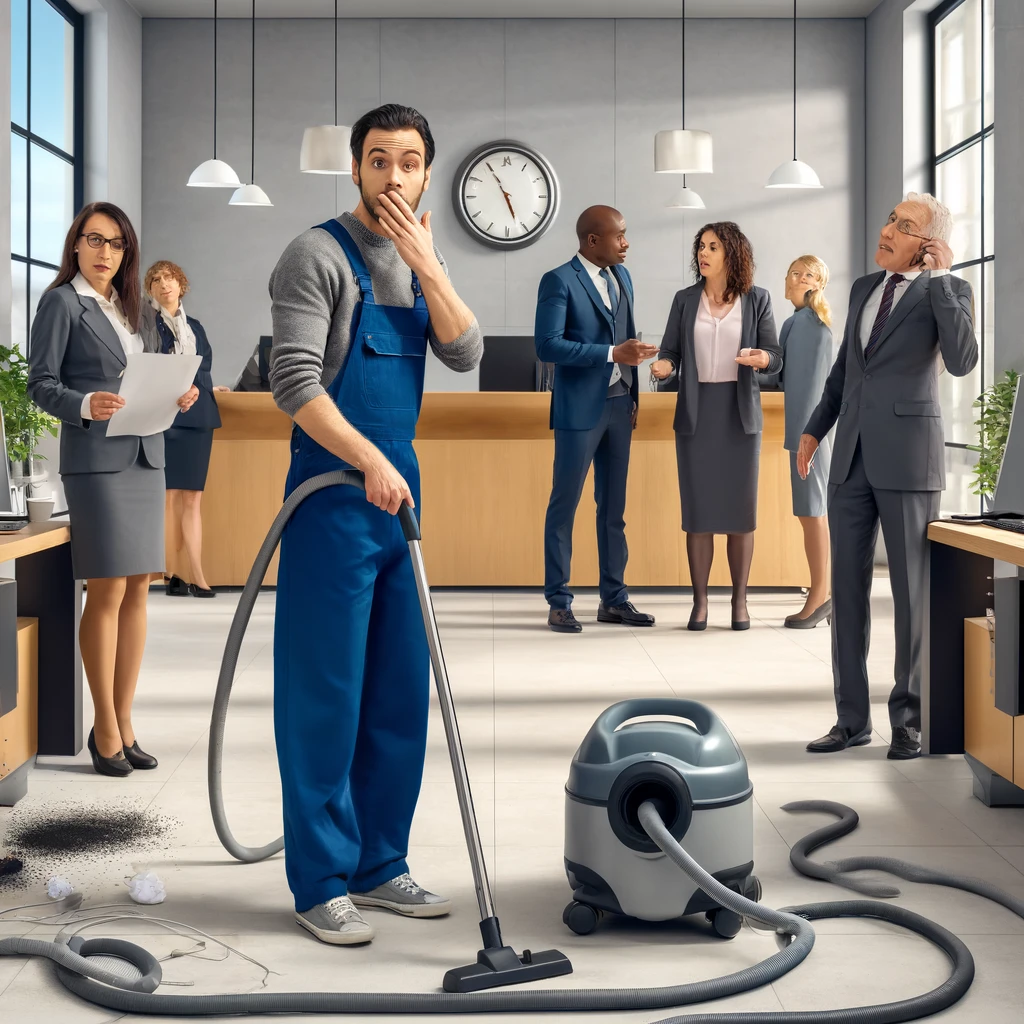
1. Downtime and Transaction Volumes: A Growing Backlog
At first, the downtime seems manageable. The average modeled downtime is 6 hours, but in more severe cases, it could last up to 18 hours or even 37 hours. As each hour passes, the number of transactions requiring AML review builds up.
Under normal conditions, the bank processes 200 transactions per hour. In a severe but plausible 36-hour outage scenario, the simulation suggests an average of 160 transactions over £2,000 will need semi-automatic processing and in an extreme event – such as an extended outage in the run up to a national holiday – this number could climb to 660 transactions. Meanwhile, while the simulation suggests on average there will be 31 high-value transactions sent for manual review, this number could rise to 140 transactions in extreme situations.
As these high-value transactions wait for manual review, customers grow impatient. Each delay compounds the risk of compensation claims and customer dissatisfaction.
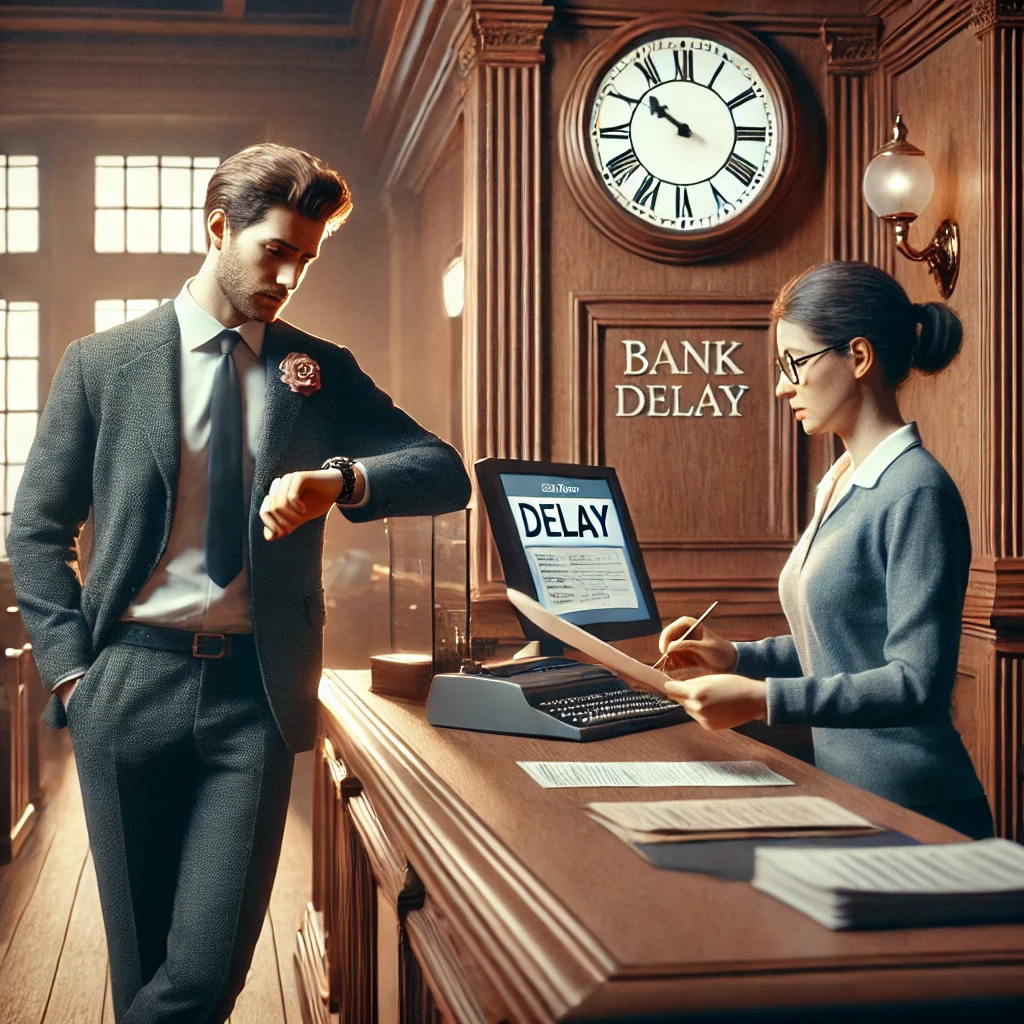
2. Compensation Costs: How Delays Add Up
Every delayed transaction carries a potential compensation cost. For mid-range transactions between £2,000 and £10,000, the bank expects to pay £100 goodwill for each delayed transaction. For high-value transactions exceeding £10,000, the compensation rises to £500 per transaction.
The simulation estimates that, on average, the compensation for mid-range transactions will amount to £13,000, however this could surge to £54,000. When high-value transactions are added to the mix, compensation costs increase further. On average, these would add £16,000 to the total, but in a worst-case scenario, this could climb to £66,000. Altogether, the total compensation costs could range from £29,000 on average, up to £120,000 in a worst-case scenario. These costs, while significant, only tell part of the story.
3. Manual Errors: An Unseen Risk
As the bank turns to manual processes, another risk emerges: human error. The base assumption is that 5% of manually processed transactions will contain errors, but under pressure, this figure could rise to 7% or more.
The simulation shows that, on average, the bank could make errors in the processing of 14 transactions resulting in an additional £3,300 in additional compensation costs. However, in a worst-case scenario, with high volumes and a higher error rate, manual errors could cost the bank up to £25,000. These errors aren’t just financially costly—they further strain operational resources and damage client trust.
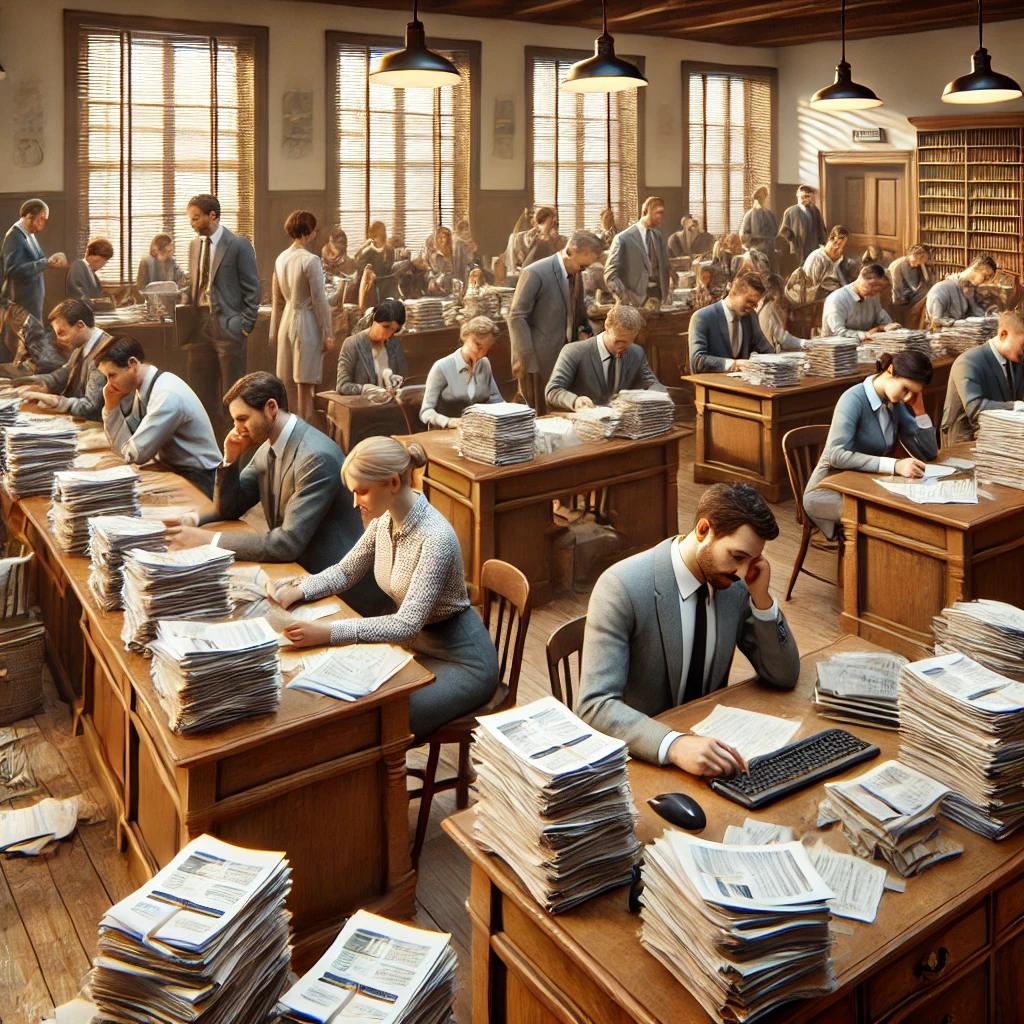
4. Worst-Case Scenario: When Everything Goes Wrong
As it turns out, the simulation suggests the event will be around 6 hours in duration, impacting around 160 customers, requiring £32,000 to be paid in compensation. However, the extreme 1-in-200 scenario, the downtime drags on, more transactions are delayed, manual errors spike, and compensation claims stack up. In this scenario, the bank would have to compensate 1,200 customers including additional payments for errors to 98 of those customers, with an expected compensation bill of £140,000. Even in a severe yet plausible 1-in-20 scenario, the compensation could still reach £87,000.
Beyond the financial impact, the reputational risk looms large. High-value clients might tolerate a short delay, but extended downtime—especially when coupled with errors—could lead to long-term damage to the bank’s customer relationships. And on top of all this, the response of the regulator could be significant.
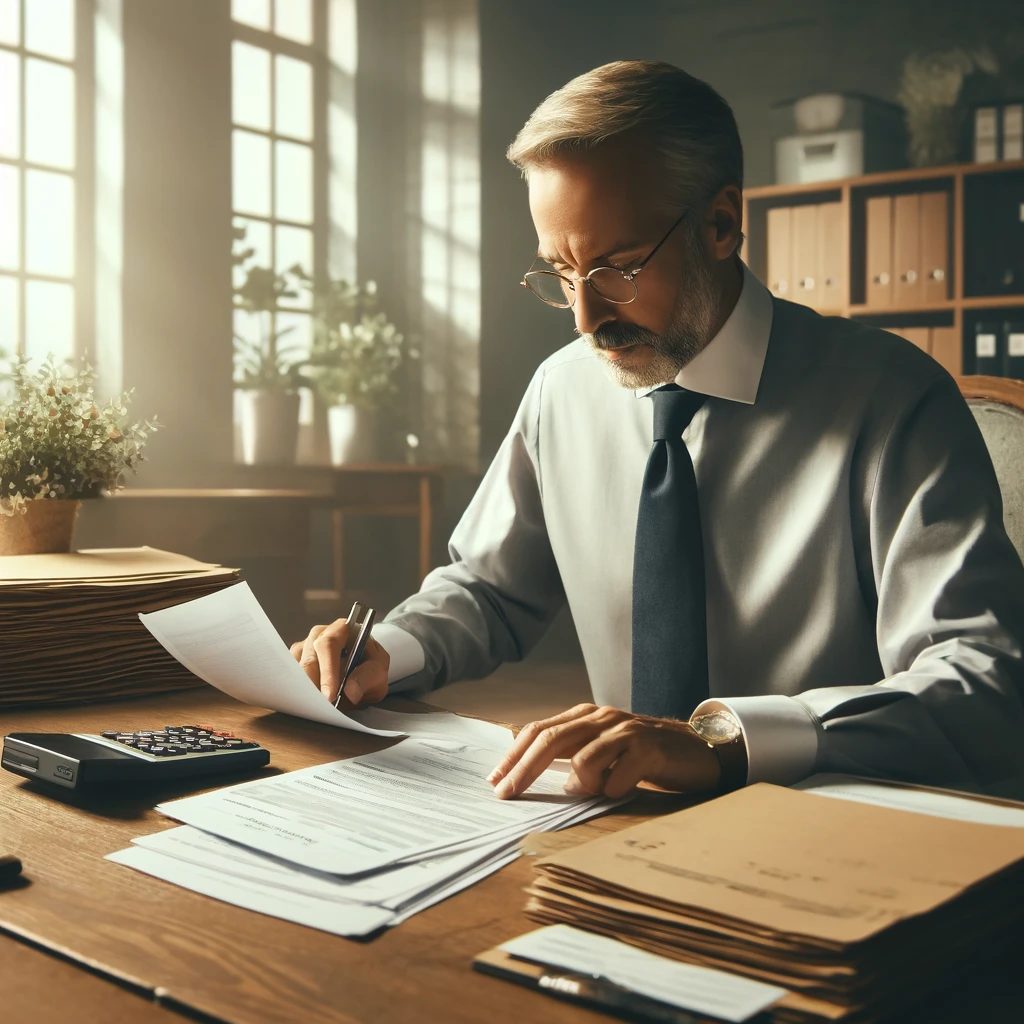
Bringing It All Together: The Broader Implications of Downtime
The narrative that emerges from this simulation isn’t just about compensation—it’s about operational vulnerability and gaining insight into our risk tolerance and thresholds. A system crash may seem like a technical glitch, but as this scenario shows, the financial and reputational risks escalate rapidly. Even with semi-automatic systems and manual reviews in place, prolonged downtime amplifies costs, frustrates customers, and risks compliance breaches.
Monte Carlo simulations give us a way to anticipate these risks, providing a clear picture of how different scenarios play out. For a bank relying on third-party services for critical AML monitoring, understanding the worst-case scenarios is essential to avoid the financial and reputational fallout.
In today’s fast-moving world, data-driven risk management is no longer optional. Firms must embrace these tools to assess operational resilience and protect against the unexpected.
Strengthen Your Operational Resilience with Simulation-Based Risk Management
In light of these findings, Risk functions should take proactive steps to incorporate Monte Carlo simulations into their operational risk management frameworks. Understanding the potential range of outcomes, from best-case to worst-case scenarios, enables better decision-making and more effective resource allocation during a crisis.
If your organisation relies on third-party services for critical functions such as AML monitoring, now is the time to evaluate your disaster recovery and business continuity plans. How well-prepared are you for a similar outage? How can simulation-based tools help quantify and mitigate these risks?
By adopting simulation-based approaches, financial institutions can better manage the complexities of operational risk and ensure they are prepared for the unexpected. In today’s uncertain world, it’s not just about managing what you know—it’s about preparing for what you don’t.
The future of risk management lies in data-driven simulations. It’s time to harness their power to secure your organisation’s financial and operational future.