A Longevity Risk Case Study
When discussing model risk in financial services, conversations often gravitate toward trading algorithms or credit scoring models. However, some of the most significant model risks lurk in longer-term business activities where validation is challenging and errors compound over years. Today, we’ll explore one such scenario through a Monte Carlo simulation of a model failure at a hypothetical specialist insurer.
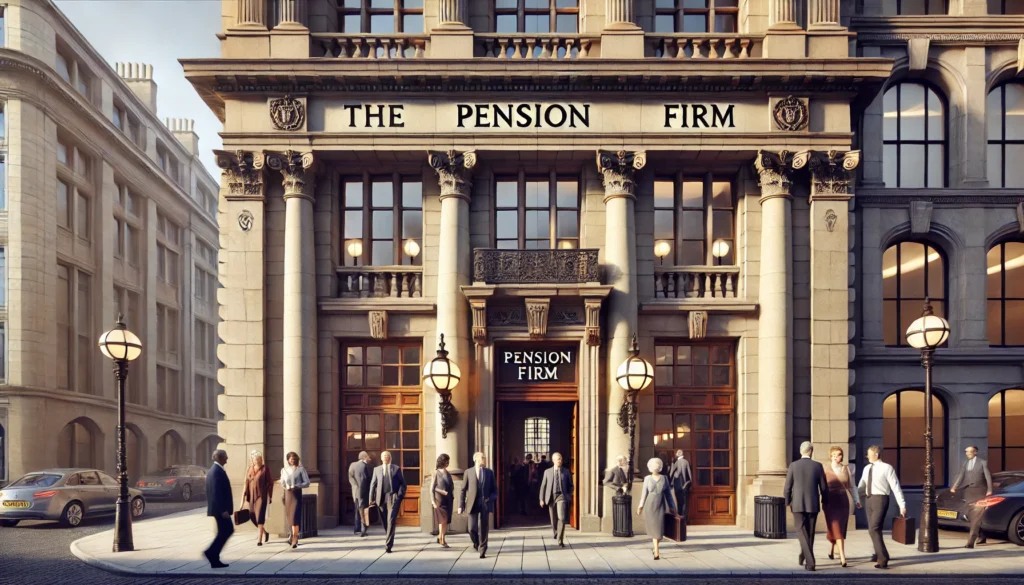
Setting the Scene: A Specialist Insurance Provider
Our case study focuses on a UK-based specialist insurer catering exclusively to high-net-worth (HNW) individuals. The firm’s unique selling proposition centres on sophisticated underwriting and bespoke pension products, particularly targeting individuals with investable assets exceeding £5 million. With approximately 400 policies on their books and average annual payments of £250,000 per policy, they manage around £100 million in annual payments – a small but focused operation in the insurance landscape.
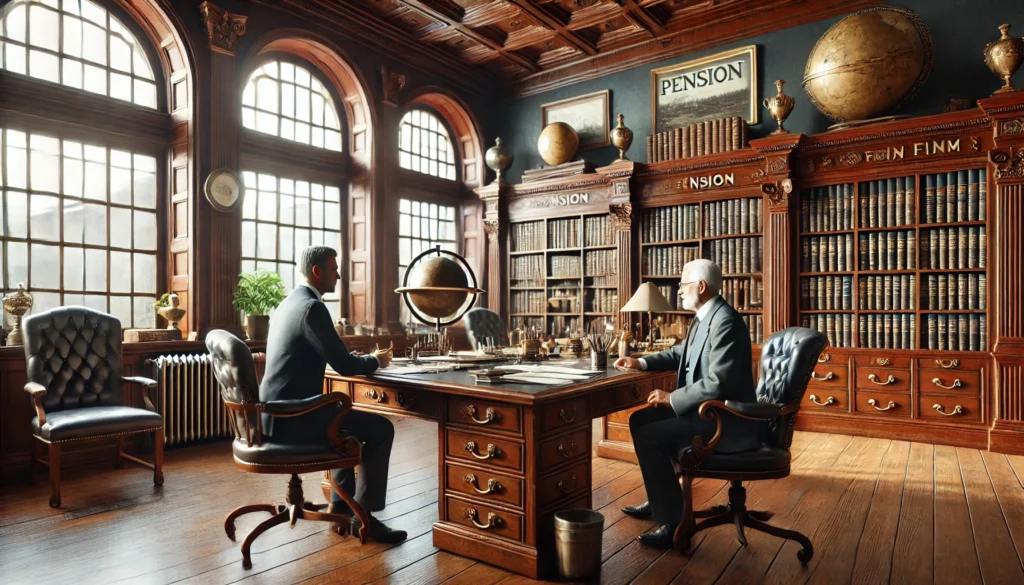
The Model Risk Scenario
The trigger event in our simulation is the discovery of a systematic underestimation in the firm’s longevity predictions for their HNW client base. The model failed to adequately capture several crucial factors affecting wealthy individuals’ life expectancy: superior access to healthcare, early adoption of life-extending treatments, and lifestyle factors specific to the HNW segment. This isn’t merely an academic concern – it directly affects the reserves needed to meet future obligations.
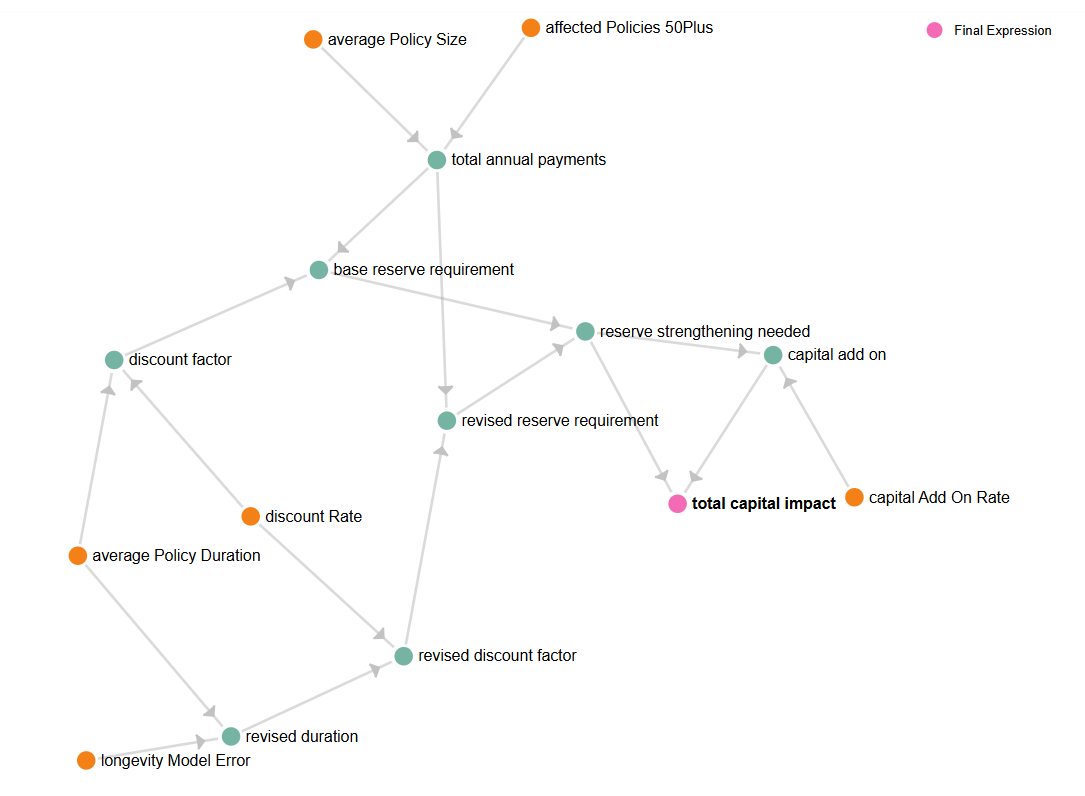
Understanding the Portfolio Characteristics
The simulation examines a portfolio of around 300 policies for clients aged 50 and above. These aren’t ordinary pension policies – with average annual payments of £250,000, they represent significant long-term commitments to wealthy individuals expecting premium service. The portfolio’s duration averages 25 years, reflecting the long-term nature of pension obligations and the relatively younger age profile of the affected clients.
Technical Impact: The Numbers Behind the Crisis
The core of our simulation revolves around the longevity model error, estimated at 15% on average. This means the model has been systematically underestimating how long clients are likely to live by about 15%. For a pension provider, this translates directly into longer payment periods and, consequently, larger reserve requirements.
The simulation calculates reserve requirements using a simplified discount rate approach, averaging 2%. This helps convert future payment obligations into today’s monetary terms. The base reserve requirement – the amount needed before discovering the model error – averages £1.47 billion. This figure makes sense given the annual payment obligations and the long-term nature of the commitments.
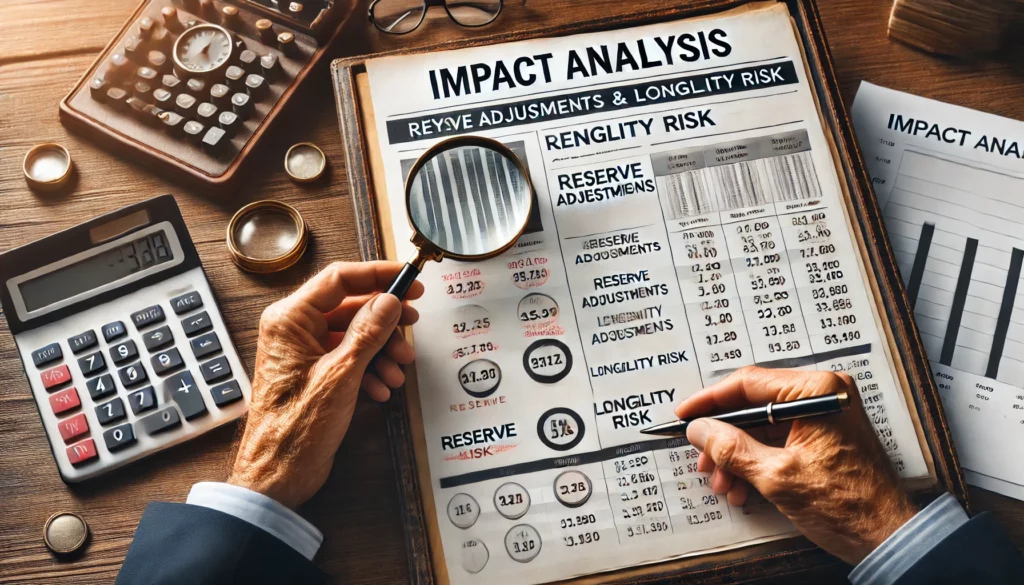
The Capital Impact
When the model error is discovered, two immediate financial impacts emerge. First, the firm needs to strengthen its reserves by approximately £161 million to account for the longer expected payment period. Second, regulators typically require additional capital (a “capital add-on”) as a buffer against uncertainty, simulated at around £24 million.
The total capital impact, averaging £187 million, represents a severe but plausible shock for an insurer of this size. To put this in perspective, it’s roughly 2.5 times the annual premium income – a significant hit that would require urgent management attention but shouldn’t necessarily be fatal to a well-capitalised specialist insurer.
Regulatory Implications
The simulation includes stress testing at levels relevant for regulatory reporting. The 1-in-20 and 1-in-200 scenarios, representing 95th and 99.5th percentiles respectively, help understand the potential severity under stressed conditions. These metrics are particularly important for the Own Risk and Solvency Assessment (ORSA) process required by insurance regulators.
The regulatory response would likely extend beyond just capital requirements. The nature of the error – systematic underestimation of longevity in a firm marketing itself on sophisticated underwriting – could trigger enhanced supervision and potentially a skilled persons review under Section 166 of the Financial Services and Markets Act.
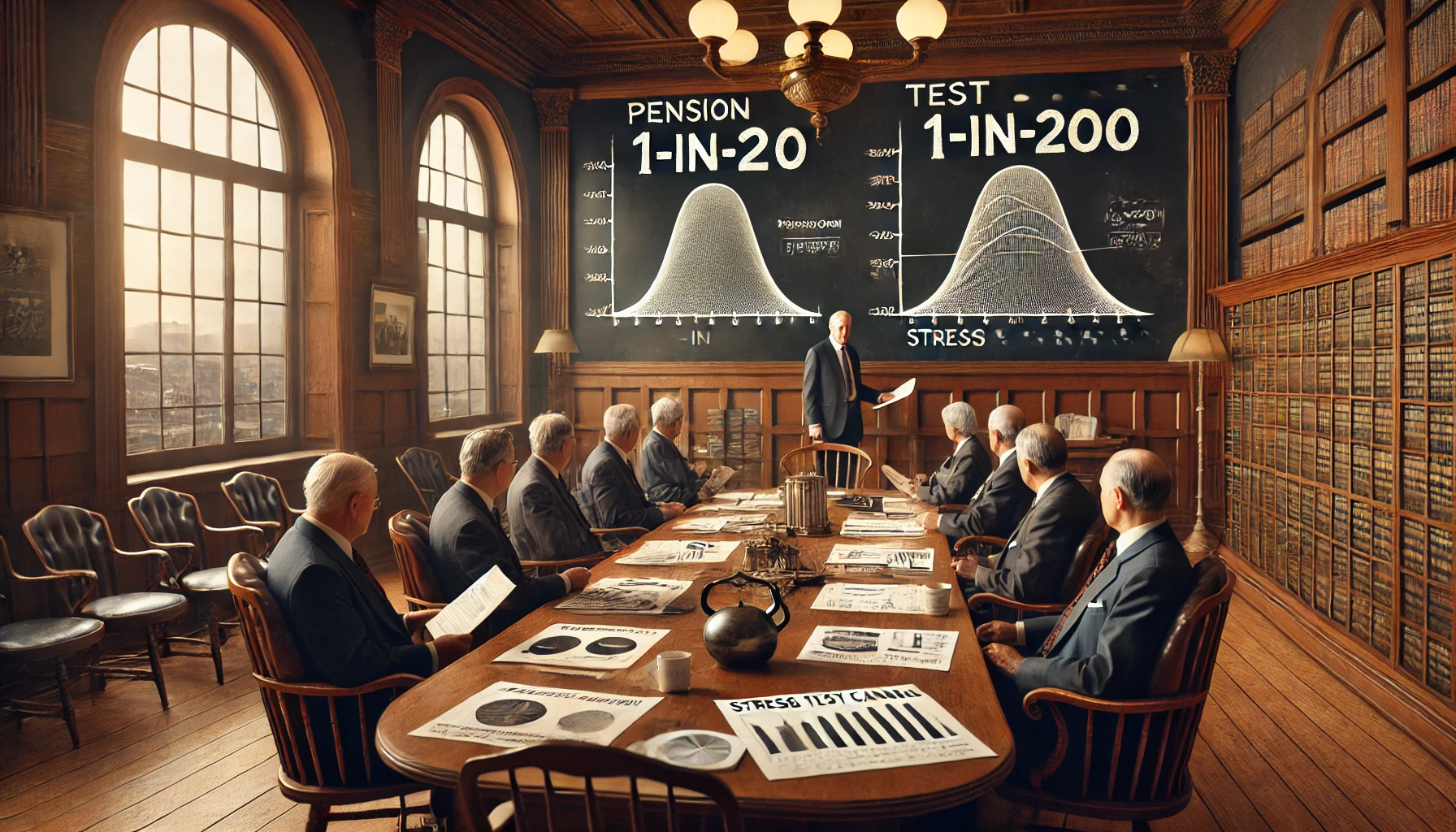
Monte Carlo Simulation Approach
The simulation employs Monte Carlo methods to model uncertainty in key parameters. Rather than using single-point estimates, we allow each parameter to vary according to specified probability distributions. This provides a more nuanced understanding of possible outcomes and their likelihood.
Key parameters include the number of affected policies, average policy size, policy duration, and the magnitude of the longevity model error. The simulation also incorporates a discount rate to properly value future cash flows. By running thousands of iterations with different combinations of these parameters, we build a comprehensive picture of potential impacts.
Interpreting the Results
The simulation results demonstrate several interesting features. The distribution of outcomes shows a clear rightward skew in the total capital impact, meaning extreme adverse scenarios are more likely than extremely favorable ones. This asymmetry makes sense given the compounding nature of longevity risk.
The mean total capital impact of £187 million represents a severe but credible scenario for a specialist insurer. It’s large enough to require significant management action – possibly including capital raising – but not so large as to be implausible for a firm of this size and specialisation.
Limitations and Considerations
While the Monte Carlo simulation provides valuable insights into the potential impact of model risk on our hypothetical specialist insurer, it’s important to acknowledge the limitations inherent in this first-pass assessment. Recognising these limitations not only adds transparency but also highlights areas for further refinement and analysis.
Magnitude of Longevity Underestimation: The simulation assumes a 15% systematic underestimation of life expectancy among high-net-worth (HNW) clients. This significant margin was deliberately chosen to illustrate the potential impact of model errors. In reality, such a substantial oversight would likely stem from multiple factors, including outdated mortality tables, failure to account for medical advancements, or misclassification of client health profiles. While plausible, this assumption underscores the need for models to be continuously updated and validated against emerging data.
Portfolio Characteristics: We assumed that 300 out of 400 policies belong to clients aged 50 and above, with an average annual payment of £250,000 per policy. These figures are estimates meant to represent a typical portfolio for a specialist insurer in the HNW segment. However, actual client demographics and policy details may vary, and more granular data would enhance the accuracy of the simulation.
Discount Rate Selection: A constant discount rate of 2% was used to calculate the present value of future payment obligations. This simplified approach doesn’t account for potential fluctuations in interest rates, inflation, or changes in the economic environment over the 25-year average policy duration. A sensitivity analysis using a range of discount rates could provide a more robust understanding of the reserve requirements under different economic scenarios.
Broader Implications for Risk Management
This case study highlights several crucial aspects of model risk management. First, model errors in long-term business can create significant exposures before detection. Second, specialist firms marketing themselves on technical expertise face amplified reputational risks from model failures. Finally, the interaction between technical errors and regulatory requirements can create compound impacts on capital.
For risk professionals outside the insurance sector, the principle remains relevant: models driving long-term business decisions require particularly robust validation and governance. The impact of model errors compounds over time, and detection may come too late for simple remediation.
The simulation also demonstrates how quantitative techniques can help risk managers understand complex scenarios. While the specific numbers matter, the real value lies in understanding the relationships between different factors and how they combine to create overall impact. This structured approach to scenario analysis can be applied across various risk types and business contexts.
Remember, while this scenario is hypothetical, it’s grounded in realistic parameters and industry experience. Similar model failures have occurred across financial services, often with comparable relative impacts. The key lesson isn’t about the specific numbers but about the importance of robust model governance, particularly for models driving long-term business decisions.